Progress in neuromorphic computing for advanced AI systems
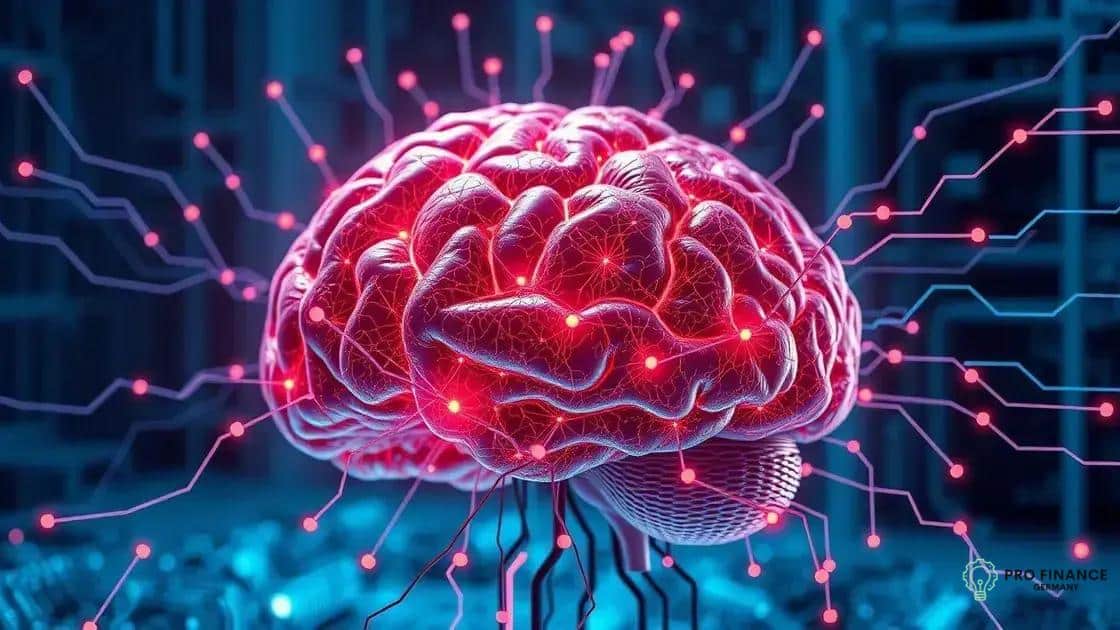
Progress in neuromorphic computing enhances AI systems by mimicking brain function for improved efficiency, real-time processing, and adaptive learning, opening new opportunities across various applications like robotics and healthcare.
Progress in neuromorphic computing for advanced AI systems is opening new doors in the world of artificial intelligence. Have you ever wondered how computing can mimic the human brain? This innovative approach not only enhances efficiency but also paves the way for more complex AI models.
Understanding neuromorphic computing
Understanding neuromorphic computing is crucial for grasping its potential in the field of artificial intelligence. This technology mimics the architecture and function of the human brain, allowing computers to process information in a more efficient manner.
Neuromorphic systems are designed to operate using large networks of artificial neurons, resembling how our brains function. These systems excel in tasks that require learning and adaptation, making them ideal for advanced AI applications.
Key Features of Neuromorphic Computing
Neuromorphic computing offers several unique features that set it apart from traditional computing approaches:
- Event-driven processing: Unlike conventional systems, neuromorphic devices react only when significant events occur, reducing energy consumption.
- Real-time learning: Neuromorphic systems can learn and adapt on-the-fly, similar to human learning processes.
- Robustness: These systems are typically more resilient to noise, allowing them to function effectively in unpredictable environments.
The adaptability of neuromorphic computing opens a range of possibilities. For instance, it enables autonomous systems to respond to their surroundings in real-time. This means robots and AI models can make decisions that are not only quick but also contextually relevant. When integrated with other AI technologies, neuromorphic systems can lead to breakthroughs in cognitive computing.
As researchers continue to explore the implications of this technology, we may see innovations that were once thought impossible. The intersection of neuromorphic computing and AI promises advancements that could redefine our understanding of machine intelligence.
Imagine a future where machines not only think but also learn and adapt as humans do. This is the vision that neuromorphic computing brings to life, paving the path for smarter, more efficient AI systems capable of complex tasks.
Key advancements in neuromorphic architectures
Key advancements in neuromorphic architectures have significantly influenced the development of intelligent systems. These innovations allow machines to process information similarly to the human brain, providing a foundation for faster and more efficient learning.
Recent breakthroughs include the creation of new materials and components specifically designed for neuromorphic computing. Advances in memristors, for example, are leading to more effective memory systems. This technology enables devices to store and process information simultaneously, mimicking synaptic connections in the brain.
Notable Features of Modern Neuromorphic Systems
Modern neuromorphic systems are characterized by several important features that enhance their functionality:
- Scalability: Recent designs allow for expanded systems that can incorporate thousands of neurons.
- Energy efficiency: With reduced power consumption, these systems perform complex tasks without draining resources.
- Real-time processing: Enhanced speed allows for immediate responses, crucial in applications like robotics and autonomous vehicles.
Another significant advancement is the use of biologically inspired circuits. These circuits improve how machines learn through experience. By modeling the way humans and animals learn from their environment, these systems become more adaptive and versatile.
As research continues, the potential applications of neuromorphic architectures grow. Their ability to function in chaotic environments makes them suitable for tasks where traditional computing struggles. For instance, they can be deployed in complex automation systems, enhancing decision-making processes in real-time.
The ongoing evolution of neuromorphic architectures will shape the future of AI technology. As these systems become more refined, we can expect a wave of innovations that further blur the lines between human cognitive functions and machine intelligence.
Applications of neuromorphic systems in AI
Applications of neuromorphic systems in AI are rapidly expanding, showcasing the versatility and efficiency of this computing approach. By mimicking the human brain’s neural structure, these systems can perform complex tasks in real-time, opening new opportunities across various industries.
One prominent application is in robotics. Neuromorphic systems enable robots to perceive their environments more effectively. This allows them to navigate, interact, and make decisions in unpredictable situations. For example, drones utilizing neuromorphic computing can process visual data instantly, improving their ability to avoid obstacles and adapt to changing conditions.
Key Areas of Application
Several key areas highlight the potential of neuromorphic systems:
- Healthcare: Neuromorphic systems can analyze medical data, aiding in patient diagnosis and treatment personalization.
- Smart homes: These systems can enhance smart home devices, improving interaction and automation based on user habits.
- Autonomous vehicles: They play a crucial role in helping vehicles understand their surroundings and make split-second decisions.
Furthermore, neuromorphic computing enhances machine learning capabilities. By processing information in a brain-like manner, these systems improve pattern recognition and data analysis. This is particularly beneficial in areas like facial recognition and natural language processing.
The adaptability of neuromorphic systems leads to more intelligent and responsive AI applications. They can learn from experiences, which allows for continuous improvement over time. As industries seek to enhance their efficiency and decision-making processes, the adoption of neuromorphic technology is likely to grow.
These advancements promise exciting developments in AI, potentially transforming how machines interact with the world. The unconventional nature of neuromorphic systems positions them as game-changers in technology, emphasizing the importance of continuing research in this cutting-edge field.
Challenges in developing neuromorphic technologies
Challenges in developing neuromorphic technologies are significant and require careful consideration. While these systems offer numerous advantages, several obstacles hinder their widespread adoption and effectiveness.
One primary challenge is the complexity of designing neuromorphic chips. These chips must precisely mimic the behavior of human neurons, which involves intricate engineering and advanced materials. This complexity often leads to longer development times and increased costs, making it difficult for companies to invest in such technologies.
Technical Limitations
Another challenge revolves around technical limitations in current neuromorphic systems:
- Limited scalability: Many existing systems struggle to scale efficiently. This prevents them from handling larger datasets or more complex tasks effectively.
- Interoperability: Integrating neuromorphic devices with traditional computing systems remains a challenge, as they often use different architectures and programming models.
- Energy consumption: Although these systems are designed to be energy-efficient, some technologies still consume more power than expected, especially when processing large amounts of data.
Developing algorithms that fully exploit the capabilities of neuromorphic hardware presents additional difficulties. Current machine learning algorithms are primarily designed for conventional architectures, requiring new approaches tailored for neuromorphic computing. This adaptation requires ongoing research and trial-and-error to achieve optimal results.
Furthermore, funding and investment in neuromorphic technology development can be inconsistent. Researchers and companies depend on grants and venture capital, which can fluctuate based on market trends and investor interest. The uncertainty can stall progress and hinder innovation in the field.
To overcome these challenges, collaboration among researchers, engineers, and industry players is crucial. By pooling expertise and resources, the development of neuromorphic technologies can accelerate. This collective effort is essential to address the existing technological hurdles and make advancements that could revolutionize AI and computing.
The future impact of neuromorphic computing on AI
The future impact of neuromorphic computing on AI is poised to be transformative. As this technology continues to advance, it may redefine the very foundations of how we perceive and implement artificial intelligence.
One significant area of impact is in enhancing the efficiency of AI algorithms. Neuromorphic systems can process information much faster than traditional computers, which means AI can analyze data in real-time. This capability leads to quicker and more accurate decisions in various applications, from healthcare diagnostics to autonomous vehicles.
Enhanced Capabilities
Neuromorphic computing will likely enable several enhanced capabilities within AI:
- Improved learning models: AI models can be designed to learn and adapt similarly to human cognition, making machines more intuitive.
- Complex problem-solving: With faster processing, neuromorphic systems can tackle complex issues that are currently beyond the reach of conventional systems.
- Greater energy efficiency: These systems are inherently more energy efficient, allowing for widespread deployment in various fields without the high costs of energy consumption.
Another important aspect is the potential for neuromorphic computing to support unsupervised learning. This refers to a type of machine learning where systems learn from data without relying solely on pre-labeled datasets. Such capabilities could lead to significant breakthroughs in AI, where machines learn from their environments and experiences similarly to humans.
As more organizations recognize the benefits of neuromorphic computing, industries such as robotics, finance, and environmental science are expected to incorporate this technology rapidly. The ability to create self-improving systems that learn and adapt will drive innovation and efficiency across various sectors.
Overall, the ongoing development of neuromorphic computing will likely influence AI in profound ways, paving the way for smarter, more adaptable systems that can solve complex problems.
FAQ – Frequently Asked Questions about Neuromorphic Computing and AI
What is neuromorphic computing?
Neuromorphic computing refers to systems designed to mimic the architecture and function of the human brain, enhancing processing speed and efficiency in AI applications.
How does neuromorphic computing improve AI performance?
By enabling real-time data processing and adaptive learning, neuromorphic computing allows AI systems to make faster and more informed decisions based on their environment.
What are some applications of neuromorphic systems?
Neuromorphic systems can be applied in various fields, including healthcare for diagnostics, robotics for navigation, and autonomous vehicles for decision-making.
What challenges exist in developing neuromorphic technologies?
Key challenges include the complexity of chip design, integrating with traditional computing systems, and creating algorithms tailored for neuromorphic hardware.